Analyzing Restaurant Reviews: Unveiling Sentimental Insights in Customer Feedback
admin
July 28, 2023
- July 28, 2023
- Case Studies, Digital Innovation, machine learning
- By admin
Analyzing Restaurant Reviews: Unveiling Sentimental Insights in Customer Feedback
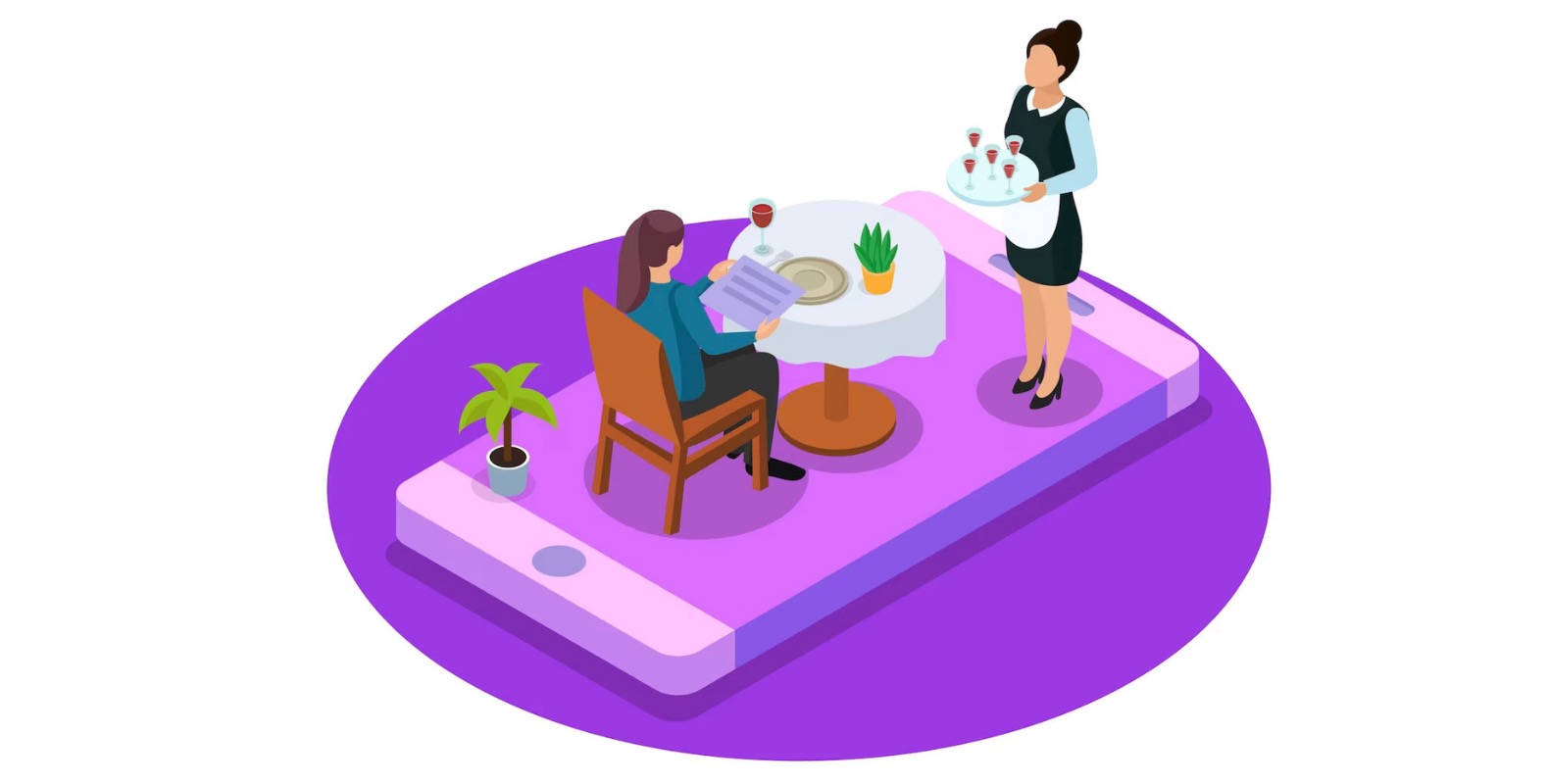
Warning: sprintf(): Too few arguments in /home/fak4qnim6k81/public_html/wp-content/themes/litho/comments.php on line 180